5 Science
Academic research is all about generating knowledge: knowledge about how the world works, and how humans operate within it. This knowledge can be desirable for its practical implications — building aircraft, developing better educational policies, curing diseases — but it can also be desirable for its own sake, satisfying our intrinsic human curiosity for understanding our environment.
There are many ways of generating knowledge. We might develop important insights into a historical phenomenon by reading appropriate source material and thinking about how it all fits together. We might learn about educational inequality by analysing demographic data and exam results for students around the country. We might learn about role of religion in indigenous societies by living with these societies and interviewing their members. Different kinds of knowledge demand different kinds of approaches, and there’s no one-size-fits-all solution that covers everything.
Scientific research corresponds to a particular approach to knowledge generation. This approach is characterised by sets of ideals about (a) what theories should look like, (b) what evidence should look like, and (c) what methods should be used to collect this evidence.
5.1 Theories
A scientific theory is a statement about how something works. This could mean all kinds of things: the way stars move through the sky, the way ants communicate with one another, or the way continents were formed.
Four criteria are held to be particularly important for scientific theories: generality, predictivity, simplicity, and reductivity.
Generality. A theory is general if it applies in a broad range of contexts and explains a broad range of phenomena. Scientists value general theories because they tend to identify more fundamental aspects of nature, as well as having more widespread practical applications. For example, a scientist would be more interested in developing a general theory of melodic patterns in baroque music than developing a theory of melodic patterns in a specific sonata by Bach.
Predictivity. A theory is predictive if it accurately predicts observed data. This predictivity is particularly valuable in situations where other theories fail to predict the same data. Predictivity is valued because it suggests that the theory provides an accurate model of the world, and because predictive theories are useful for real-world decision-making (e.g. how to combat inflation, how to improve educational attainment in inner cities, how to counteract global heating). A prerequisite for a theory to be predictive is for it to be testable, which means generating predictions that can one day be evaluated against scientific observations. Scientists have little time for untestable theories because they have no chance of satisfying the predictivity criterion.
Simplicity. Scientists value simple theories over complex theories, a principle which is often termed Occam’s razor. This principle has practical advantages: it makes theories easier to reason about, easier to test, and easier to communicate to others. There are also some deep mathematical reasons why one should, all else aside, prefer simpler theories (e.g. Chapter 28 of (MacKay, 2003)). The clinching motivation for Occam’s razor, though, is simply that it seems to work very well in practice. One famous example is when the mathematician and astronomer Nicolaus Copernicus (1473-1543) proposed his heliocentric model which placed the Sun at the centre of the universe, as opposed to Ptolemy’s geocentric model which placed the Earth at the centre of the universe. Copernicus’s new model was no better at predicting the movements of celestial bodies than contemporary geocentric models, but it was much simpler, and hence favoured by Occam’s razor. Ultimately, of course, Copernicus’s model proved to be a much more accurate description of the universe (or at least of our solar system!).
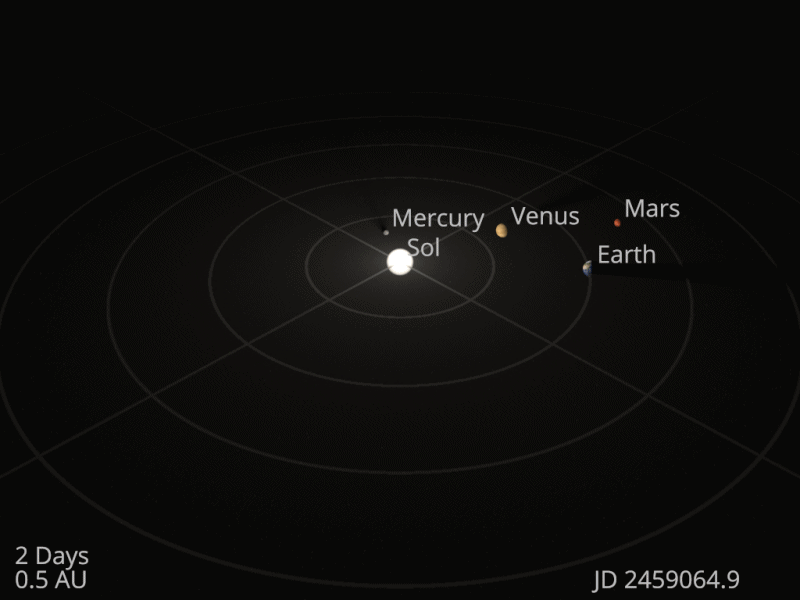
Inner planets of the solar system. Credit: Datumizer, CC BY-SA 4.0, via Wikimedia Commons
Reductivity. Scientists believe that all natural phenomena can ultimately be explained by breaking them down into their constituent parts. For example, the social dynamics studied by sociologists will reflect psychological aspects of the individuals in the social group; these psychological aspects have their basis in the behaviour of individual neurons within the brain; the behaviour of these neurons is driven by chemical reactions; these chemical reactions are a consequence of the basic physics of atoms and molecules. Scientific theories must fit coherently within this reductionist framework.
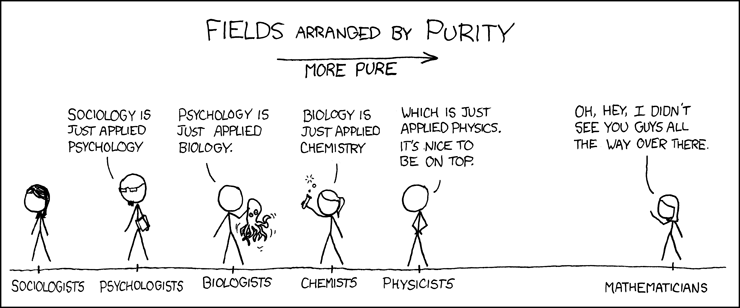
Credit: XKCD, CC BY-NC 2.5
5.2 Evidence
Scientists rely on evidence for formulating and evaluating their theories. Three criteria are particularly valued for scientific evidence: objectivity, replicability, and theoretical impact.
Objectivity. A piece of evidence is objective if it is independent of the subjective biases of the researcher. Conversely, a piece of evidence is subjective if it depends substantially on a researcher’s personal judgements, feelings or experiences. For example, if I wanted to talk about changes in chord progressions through the history of jazz harmony, it would not be very objective to say “I listened to a lot of jazz music over the weekend, and I noticed that older jazz tends to use perfect-fourth root progressions much more than recent jazz”. It would be much more objective to say “I used a computer to count root progressions in a dataset of 1,000 jazz standards, and I found a statistically reliable decrease in perfect-fourth root progressions over time” (see (Broze & Shanahan, 2013) for such an analysis).
Replicability. A piece of evidence is replicable if it can be recreated by other researchers using analogous methods. If a piece of evidence proves not to be replicable this suggests that the original result may have been a statistical fluke, or that the initial methods were flawed in an unexpected way. In either case, non-replicable results are poor building blocks for scientific theories.
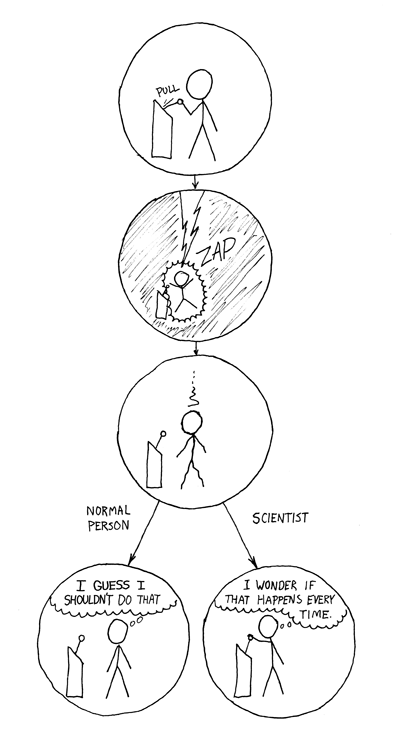
Credit: XKCD, CC BY-NC 2.5
Theoretical impact. A piece of evidence has high theoretical impact to the extent that it helps (a) to distinguish between competing scientific theories, or (b) to generate new scientific theories. One exciting case is where the research community has a built a strong consensus around a particular theory, but then a new piece of evidence demonstrates that the theory is fundamentally flawed. An alternative exciting case is when a new theory makes a special and unexpected prediction that is subsequently confirmed by a new piece of evidence. One such example is when Albert Einstein predicted the existence of gravitational waves – ripples in spacetime – on the basis of his general theory of relativity (Einstein, 1918).
5.3 Methodology
The scientific method is further distinguished by the methodologies that scientists use for generating the evidence that supports their theories.
Measurement. The process of generating scientific evidence begins with measurement. To measure something means to perform an observation according to a standardised procedure that returns a basic piece of data such as a number (e.g. 4.7), a collection of numbers (e.g. [4.7, 2.2, 1.5]), or a category (e.g. ‘red’). The purpose of this standardisation is to maximise objectivity, by minimising the subjective role of the experimenter who constructs the results.
Quantitative analysis. In order to generate meaningful insights from measured data, scientists rely heavily on quantitative analysis techniques. These are analysis techniques that take basic pieces of data (e.g. numbers, categories), and return summaries of this data, ranging from basic descriptive statistics (e.g. “the mean observed value was 5.4”) to advanced relational statistics (e.g. “the estimated causal effect of X on Y is 4.2”). Importantly, these analysis techniques are designed to be objective, in that they minimise the subjective role of the experimenter who interprets the results. Instead of relying on intuitions gleaned from examining the data ‘manually’, these methods rely on mathematical theories of probability and statistics, providing standardised analysis procedures that could be followed by any quantitative researcher.1
References
Broze, Y., & Shanahan, D. (2013). Diachronic changes in jazz harmony: A cognitive perspective. Music Perception, 31(1), 32–45. https://doi.org/10.1525/rep.2008.104.1.92
Einstein, A. (1918). Über gravitationswellen. Sitzungsberichte Der Königlich Preußischen Akademie Der Wissenschaften, 154–167.
MacKay, D. J. C. (2003). Information theory, inference and learning algorithms. Cambridge University Press.